Leveraging combinations of epigenomic regulators
authors
keywords
- Epigenomic regulators
- Combinations
- Machine learning
- Cis-Regulatory Elements
- Autoencoders
- Statistical modeling
- Monte Carlo
document type
THESEabstract
Genetic cis-regulation in humans is effected through chromatin regulators, such as histone marks and Transcriptional Regulators (TRs), binding on regions called Cis-Regulatory Elements. Those regulators seldom act alone, forming complexes to perform their functions. For example, while Transcription Factors are regulatory proteins that bind directly to DNA, they are themselves bound by co-factors. The goal of these interacting systems is to regulate gene expression by influencing the activity of the RNA Pol II, which transcribes DNA to messenger RNA. The development of Next Generation Sequencing provides experimental methods to study this regulation, which includes ChIP-seq and other assays. Their main goal is to quantify both chromatin accessibility and protein binding. However, these methods present challenges and sources of noise, where noise is defined as any result differing from the biological reality being quantified. They also suffer from reproducibility problems, hence complicating fair comparison among results. Both these biases are difficult to correct. Besides combinations of regulators themselves, the recent explosion of available data volume, as well as variety of sources, collated in databases such as ENCODE or ReMap gives opportunities for integrating different data views. While combinations of biological regulators are important to genomic cis-regulation, they are seldom operated for biological insight. Existing approaches suffer from either the precision of the data integration, or the clarity of usage. The goal of this thesis is to leverage such combinations through the use of machine learning methods, which are very effective at learning regularities in the data: in other words, learning combinations. We propose to represent the regions where regulators bind as lists of intervals, converted into matrix and tensor representations. As a result, the approaches of this thesis are generalizable to any lists of intervals. Early work presented in this thesis discusses the prediction of cis-regulatory region status and the detection of alternative promoters in T-ALL leukemia. We propose a new method, based on Cramer’s V-score, to robustly identify meaningful alternative promoters in based on promoter expression, discarding low-level noise. Then, we focus on anomaly detection. ChIP-seq and other experimental assays can suffer from errors and false positives, poor quality control, and several other biases. Those are very difficult to correct, as annotated supervised data is rarely available, and even so it would require a tedious error-by-error approach. Furthermore, the indiscriminate use of larger volumes of data increases the probability of erroneous observations. Instead, we perform unsupervised anomaly detection under the assumption that noise peaks will not respect the usual combinations between sources (ie. combinations between regulators and/or usual dataset combinations). We propose the atyPeak method which exploits not only combinations of TRs, but also combinations of redundant experiments from the ReMap database. We propose to use a specifically designed multi-view convolutional autoencoder to perform a “Goldilocks” compression. Here, the model is tasked to learn sources (TR, datasets) as part of a groups of correlating sources and not alone. As a result, ChIP-Seq peaks are rebuild as part of a correlation group and rare noisy patterns are not even learned. We identify peaks which have fewer known collaborators present in their vicinity than what would be average for their sources. In terms of methodology, we developed approaches to evaluate autoencoders based on their respect of existing correlations. We also propose a new normalization method based on correcting for the average cardinality of the aforementioned correlation groups. It can be applied to any black box model, and is useful to interpret autoencoders when performing anomaly detection. Our cleaned data improves Cis-Regulatory Element detection. Finally, on a more fundamental level, the enrichment of given combinations of elements (meaninghow much more often they are found compared to expected by chance) needs to be precisely quantified. We propose the OLOGRAM-MODL approach, demonstrating a Monte Carlo based method to fit a novel Negative Binomial model on the number of base pairs on which a given combinations of elements is observed. This allows us to return much more precise p-values compared to existing approaches. We extend this model to combinations of any k ≥ 2 elements. We also propose a suited itemset mining algorithm to identify interesting combinations of regulators, based on which itemsets best rebuild the original data. This algorithm leverages dictionary learning for its robustness to noise. Additionally, we demonstrate that the problem is submodular and that a greedy algorithm can find itemsets of interest. This tool was implemented as a part of the gtftk toolset for ease of access.
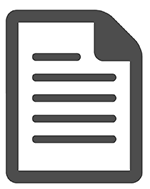